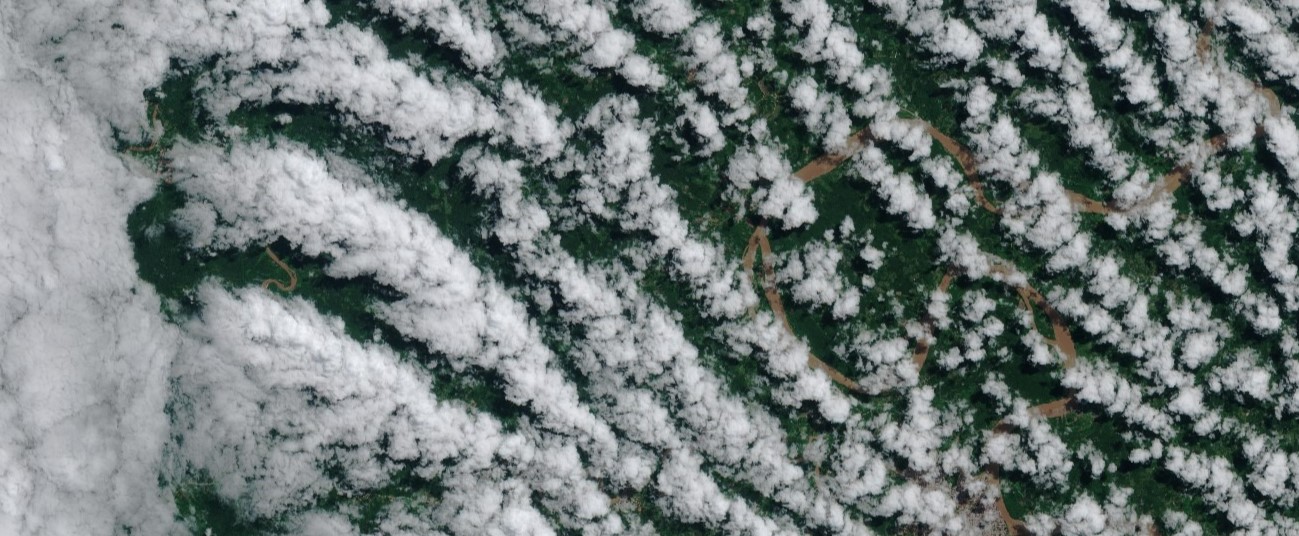
This data story is part of a series on new satellite-based data about deforestation and land use changes in the Peruvian Amazon developed through the European Space Agency's (ESA) Global Development Assistance (GDA) program under a partnership between ESA and the World Bank.
Forests play a crucial role in Earth’s carbon cycle as they absorb and store large amounts of carbon from the atmosphere. However, climate change, forest degradation and deforestation are causing much of this stored carbon to be released back into the atmosphere, particularly in tropical humid regions. To reverse this trend, financial investments are essential for sustainable forest management, restoring degraded and deforested lands, and supporting conservation efforts that protect these vital carbon sinks. Investors and standard setters increasingly demand measurable impact for green investments — but this isn't always easy, especially when data limitations have been a bottleneck. This data story discusses how cloud-penetrating data can help overcome these challenges, providing more reliable and frequent insights to track progress and ensure accountability.
For countries to reduce greenhouse gas (GHG) emissions, keeping forests intact is critical. The Peru Country Climate and Development Report (CCDR) notes the importance of tackling deforestation, as it is the country’s main source of GHG emissions. 53 percent of emissions are due to land cover changes in forested areas, also known as Land Use, Land Use-Change and Forestry (LULUCF), of which 85 percent stem from agricultural activities. Protecting existing tree cover and growing new forests are important mechanisms for durable carbon sequestration through the capture capabilities of biomass and forest soils.
High-resolution data on land cover transitions are needed to track forest disturbances and to monitor when, where and how these changes occur. Since the data from Hansen et al. (2013) was released, it has been widely used and provides annual, country-wide numbers. With the new dataset presented here, we raise the spatial and temporal resolution of land cover data to better monitor forest-related policies.
The "how" and "why" of forest cover changes
In answering the first question — the how — measurement is key but obfuscated by significant challenges. The scarcity of timely and reliable data on deforestation has imposed limitations on policy actions. The availability of data in terms of frequency, time span, sensing capability, and spatial resolution, can widely vary. Particularly, cloud cover is one of the main challenges that tropical forests, such as the Amazon, pose, which has long prevented continuous monitoring strategies and data collection using remote sensing.
Cloud cover can block optical satellites from seeing the Earth's surface, reducing the amount of data they can gather. In the Amazon basin, clouds are present for much of the year. Since satellite data used to monitor deforestation requires a clear view to be accurate, cloud cover has traditionally caused gaps in the data collected throughout the year. To address this, optical remote sensing combines clear sections of multiple satellite images over a period of time to create "mosaics." While this method has made it possible to produce reliable yearly updates, gathering detailed data at more frequent intervals has been a challenge.
New techniques combining time series fitting and segmentation have emerged to challenge the dominance of mosaicking techniques, offering a rich description of land cover characteristics and a within-year change detection. Furthermore, availability of Synthetic Aperture Radar (SAR) remote sensing technology allows image collection independent of atmospheric conditions, daylight, or weather. These sensors generate their own illumination in wavelengths where clouds are almost fully transparent, enabling image acquisitions in both nighttime and cloudy conditions.
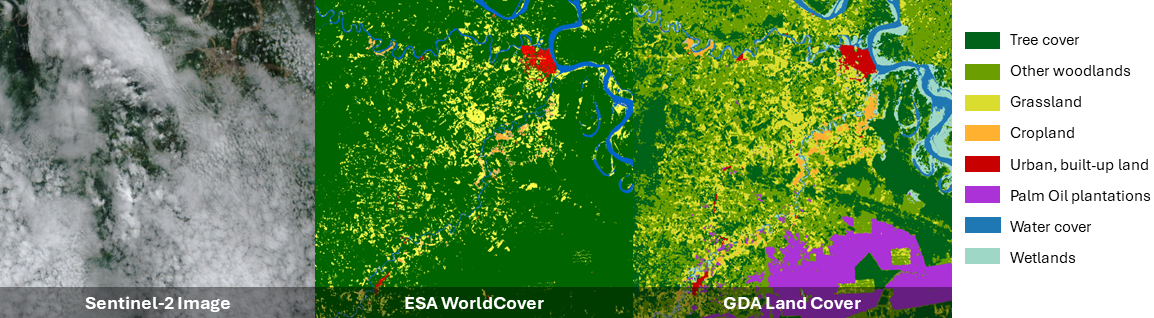
In answering the second question — the why — land use and land cover [1] transitions are essential for understanding deforestation, as it offers us a way of linking the phenomenon to the intrinsic factors that motivated the change. Taking advantage of ESA’s Climate Change Initiative (CCI) project, the ESG Data Portal´s Landcover Transition Tool uses land cover information to understand what motivated deforestation. These numbers are based on a spatial resolution of 300 meters and a yearly frequency between 1985 and 2022. The new data product raises the spatial resolution to 30 meters, all the possible transitions in and out of forests, from one period to another as in the graph below.
Detecting land cover changes is possible within this framework due to the use of the Continuous Change Detection and Classification (CCDC) algorithm, which allows us to analyze the behavior of each pixel of satellite-based images over time and identify the times at which each pixel’s behavior changed. This methodology enables change detection within a temporal context, which will also helps account for seasonality and time series variation. If this algorithm detects any abrupt change in the behavior of a pixel, it will be associated with a new land cover class.
The biannual land cover maps from 1985 to 2017 have been generated with Landsat imagery following the Continuous Change Detection and Classification (CCDC) methodology. The rationale behind this workflow is that spectral information contained in single images may not be enough to separate land use and land cover. For example, tilled croplands and bare soil will look similar during some parts of the year, but they can be separated by their temporal behaviour: crops have a green-up period, whereas bare areas do not change. CCDC performs classification based on a group of functions fitted to describe the temporal behaviour of each instrument band. In other words, it is based on the spectral-temporal signature of land cover.
Regular image acquisitions from Sentinel-1 over our region of study became available in 2017, making it possible to create a fit based on SAR data. This allowed to perform classification in cases where there was no Landsat fit available, or to enrich the feature set by combining Landsat and Sentinel-1, reducing uncertainty. It should be noted that SAR imagery has its own limitations, such as radiometric distortions appearing over steep slopes. These areas have been masked in Sentinel-1 fits; case where only Landsat fits were used for classification.
All CCDC results (Landsat, Sentinel-1 and combined) are used by a machine learning classifier algorithm to predict the IPCC land cover classes over the area of interest.
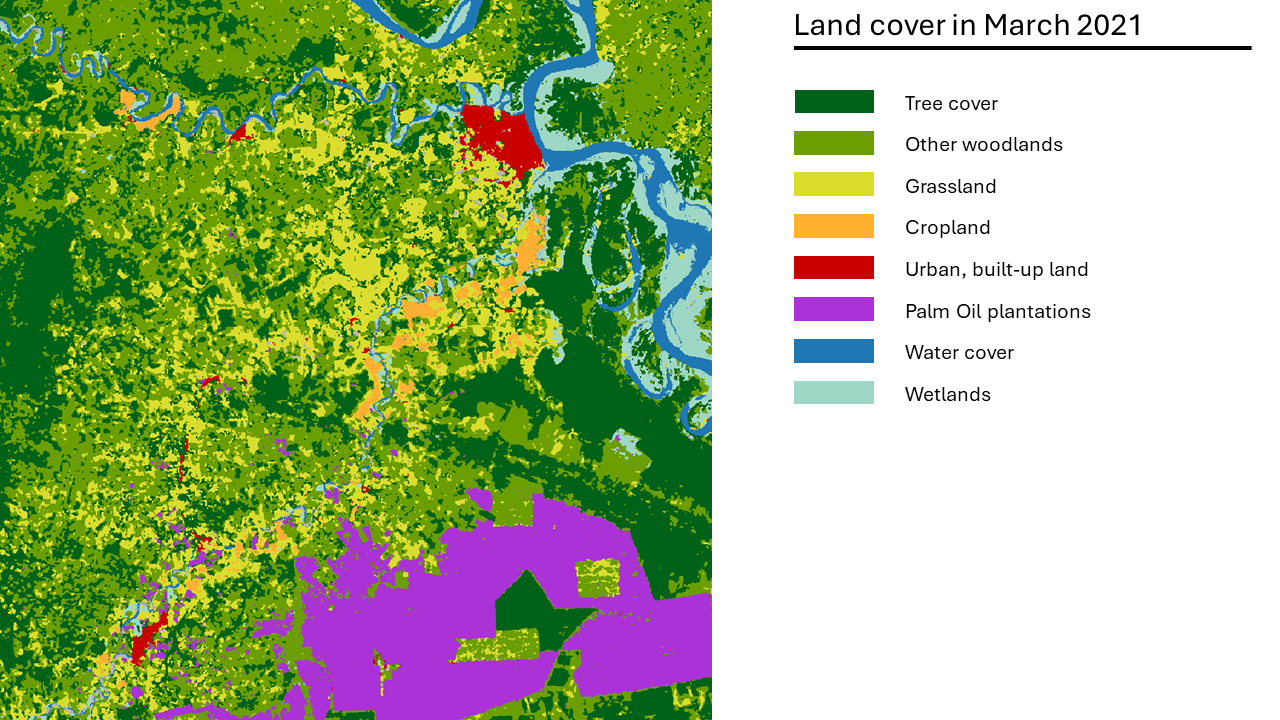
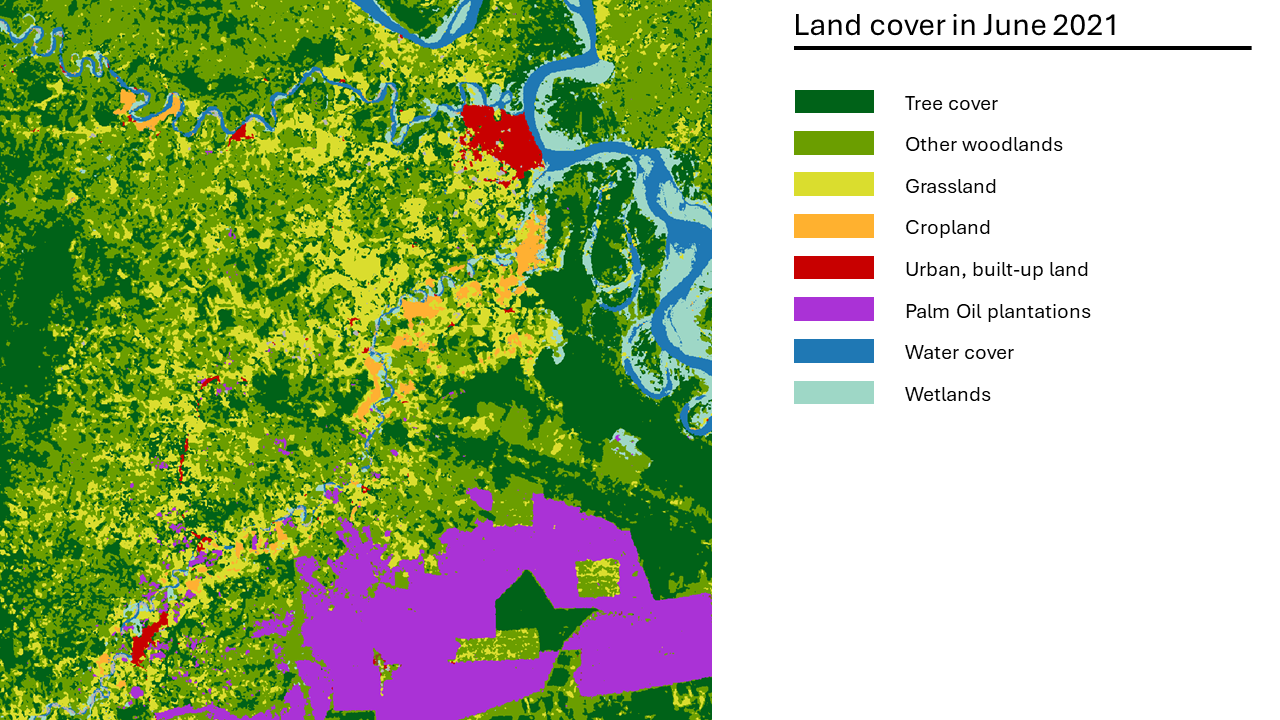
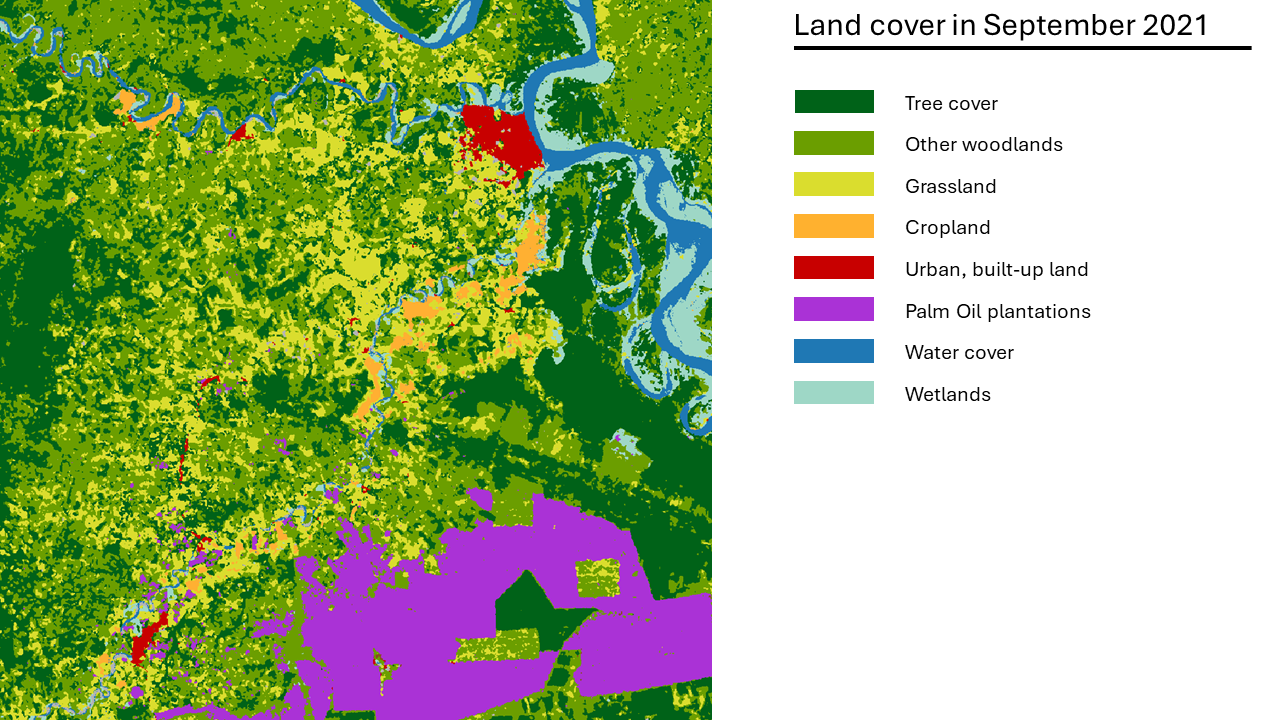

A deeper dive into the Peruvian Amazon
The Peruvian Amazon is the second-largest swath of the Amazon, after the Brazilian Amazon, and covers nearly two-thirds of Peru’s total land area. It is the home to almost 4,000 unique and threatened species as well as hundreds of indigenous communities. The Peruvian Amazon is also vital in the global fight against climate change as one of our planet’s most important carbon storages, containing over 48.2 billion metric tons of carbon.
The combination of the CCDC algorithm and SAR imagery created reliable, sub-annual maps of deforestation and land use in the Peruvian rainforest. Through the partnership between the World Bank and the European Space Agency under the GDA, new information on historical deforestation hotspots is available sub-annually (1985-2016) and quarterly (2017-2022).
An example use case is that of Yurimaguas, a historically known deforestation hotspot located in the Loreto region of the Peruvian Amazon where mechanized agriculture and legal logging are taking place. Without a doubt, land conversion has occurred at the cost of forests.
LULUCF in Peru
- Landsat 5, 7 and 8
- Sentinel-1
Background
Current annual land cover maps for Yurimaguas are generated using satellite optical imagery (Landsat 5, 7 and 8, and Sentinel-1). For every year, two multi-spectral composites from rainy and dry seasons are taken to consider land cover temporal signatures. A random forest is used to classify every pixel based on the spectral values at these two moments, producing class probability estimates as an independent output. These probabilities can help users to navigate transition uncertainty and identify conflicts between the classes involved.
The use of synthetic aperture radar (SAR) satellite instruments, capable of imaging the ground regardless of sun illumination or cloud cover is expected for the next update. This technology can provide a detailed description of the land cover temporal signature, more informative than two yearly slices, being able to inform on the sub-annual changes. This temporal trajectory is leveraged using the continuous change detection and classification framework (CCDC). CCDC allows to detect forest loss as breaks in the temporal trajectory, and to classify the post-change land cover based on the new temporal trajectory. Furthermore, CCDC can be applied to optical imagery, helping to remove spurious change alerts caused by clouds or shadows. Stay tuned for more information on how we approach it.
This new data product is not only valuable for conservation purposes and tracking forests over time and space. Part 2 of the data story (coming soon) discusses how we can leverage this type of data to structure innovative financing products and design reliable monitoring systems.