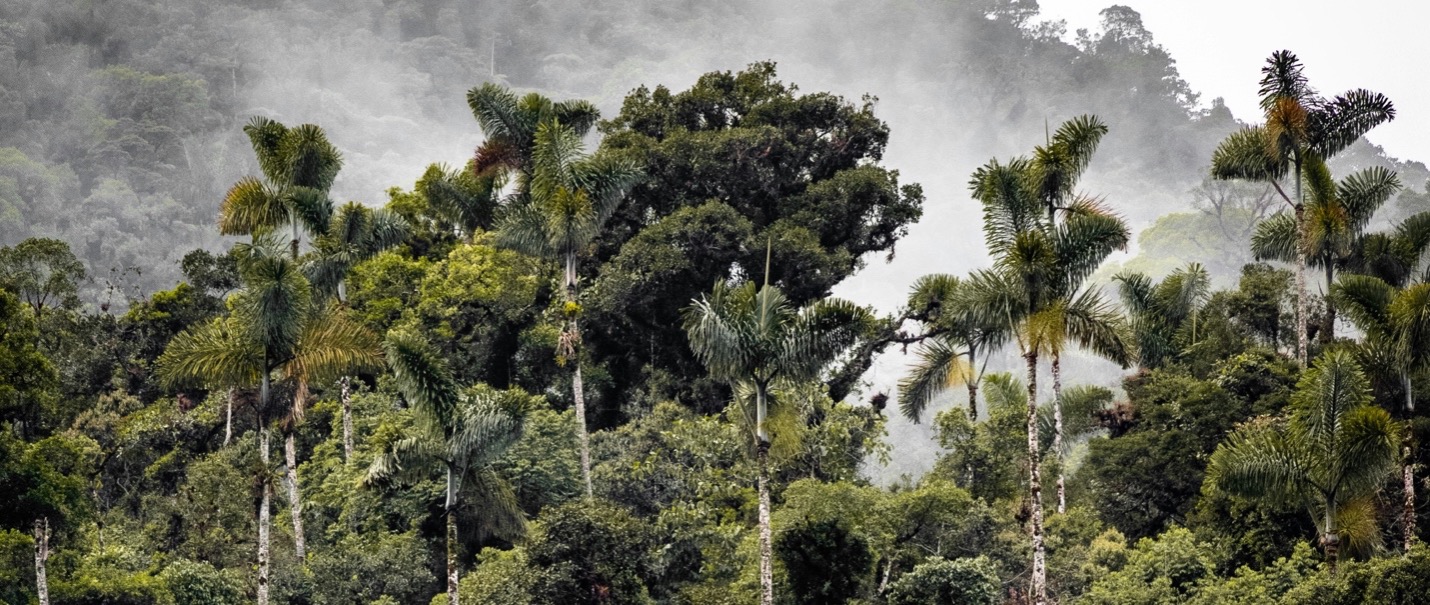
This data story is the second part of a series on new satellite-based data about deforestation and land use changes in the Peruvian Amazon developed through the European Space Agency's (ESA) Global Development Assistance (GDA) program under a partnership between ESA and the World Bank. Read Part 1 here: Peering through the clouds in the Peruvian Amazon.
Financial instruments that enhance sustainability are on the rise in Latin America, especially those that target forest preservation. Uruguay, for example, issued a Sovereign Sustainability-Linked Bond (SLB) with the maintenance of native forest area as one of its key performance indicators (KPIs). Together with the Inter-American Development Bank, the World Bank is developing the core elements of the guidelines for the so-called Amazonia Bonds . These innovative Green, Social and Sustainability Bonds will support the holistic, sustainable development of Amazonia and finance zero deforestation efforts in the Amazon region.
Peru's green finance agenda has gained momentum recently, with the country's first Sustainable Bonds in October 2021 ($2.25 billion in 12-year bond and $1 billion in 50-year bond) under its Sustainable Bond Framework , a $1 billion social bond and a $2.5 billion sustainable sovereign bond in local currency. KPI-linked green finance, including SLBs, can be an important instrument to support Peru's green finance agenda in line with the development of climate finance instruments that Peru's Green Finance Roadmap prioritizes.
KPI-linked green finance incentivizes borrowers to achieve ambitious, predetermined sustainability performance targets by linking financial incentives to sustainability performance. Well-defined KPIs are necessary to generate credible, reliable and transparent measures of progress towards the sustainability objectives (Flugge, Mok, Stewart 2021) .
The updated Hansen et al. (2013) dataset made available by Global Forest Watch is a commonly used source for tree cover loss data at the national level. It follows a harmonized methodology that makes the data internationally comparable. [1] However, the limitations of that dataset in terms of frequency and granularity reduce its usefulness for reporting on three crucial elements of forest preservation: sub-annual seasonality, land conversion of forest, and sub-national performance.
In the first part of this series , we introduced an improved data product for tree cover transitions using cloud peering technologies, combined with an enhanced change detection algorithm. This new data provides more nuance on the temporal and spatial dimensions of deforestation, strengthening the credibility of Monitoring, Reporting and Verification (MRV) systems. It also allows targets to be better tailored to the country specific context while remaining comparable across countries in the same geographic or climatic group.
Tracking progress with sub-annual KPIs
On a temporal dimension, we can now establish sub-annual KPIs because measurements happen more frequently, are less impacted by cloud cover and because images from different seasons are not overlayed to produce one annual mosaic image. Having information at the sub-annual level will help to set targets throughout the year, account for seasonality and weather when monitoring deforestation and compare outcomes against similar time spans.
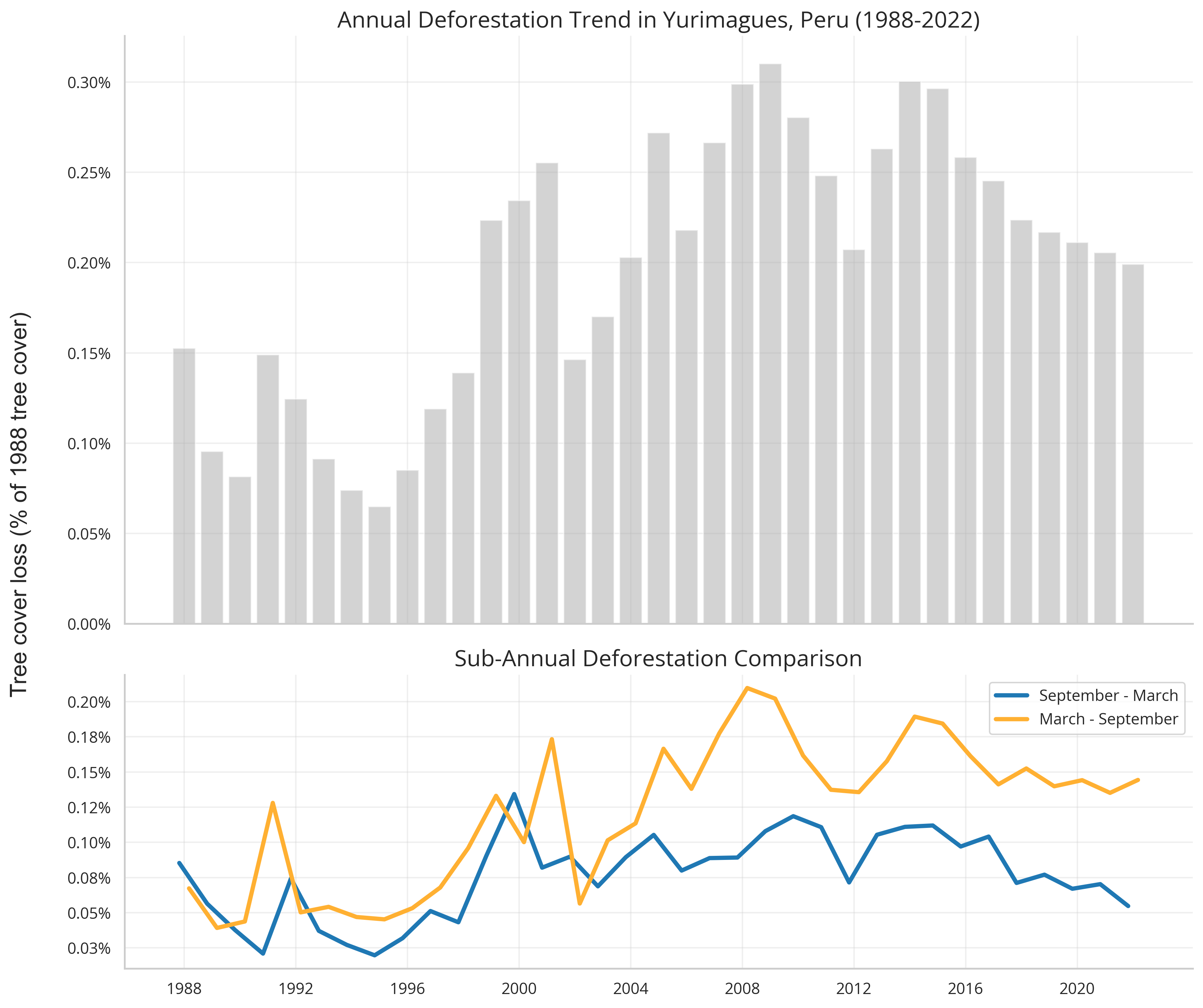
Tailoring targets to the context
New information on land cover transitions tells us what is happening now on recently deforested land. It allows us to target policies and to set targets based on land use. Deforestation in Latin America is mostly driven by agricultural demand, yet a single map of deforestation aggregates many different land-use changes into an oversimplified image. With information on land use, we can reflect the diverse landscape and learn about the economic incentives for deforestation.
In this case study, we tailored the data to the context of Peru and classified oil palm plantations as a separate land cover class. The detailed land cover transition data would allow us to target policies to the relevant drivers of deforestation and to set KPIs based on more specific objectives. For example, a general KPI on reducing deforestation could be refined to specify reducing deforestation due to palm oil expansion.
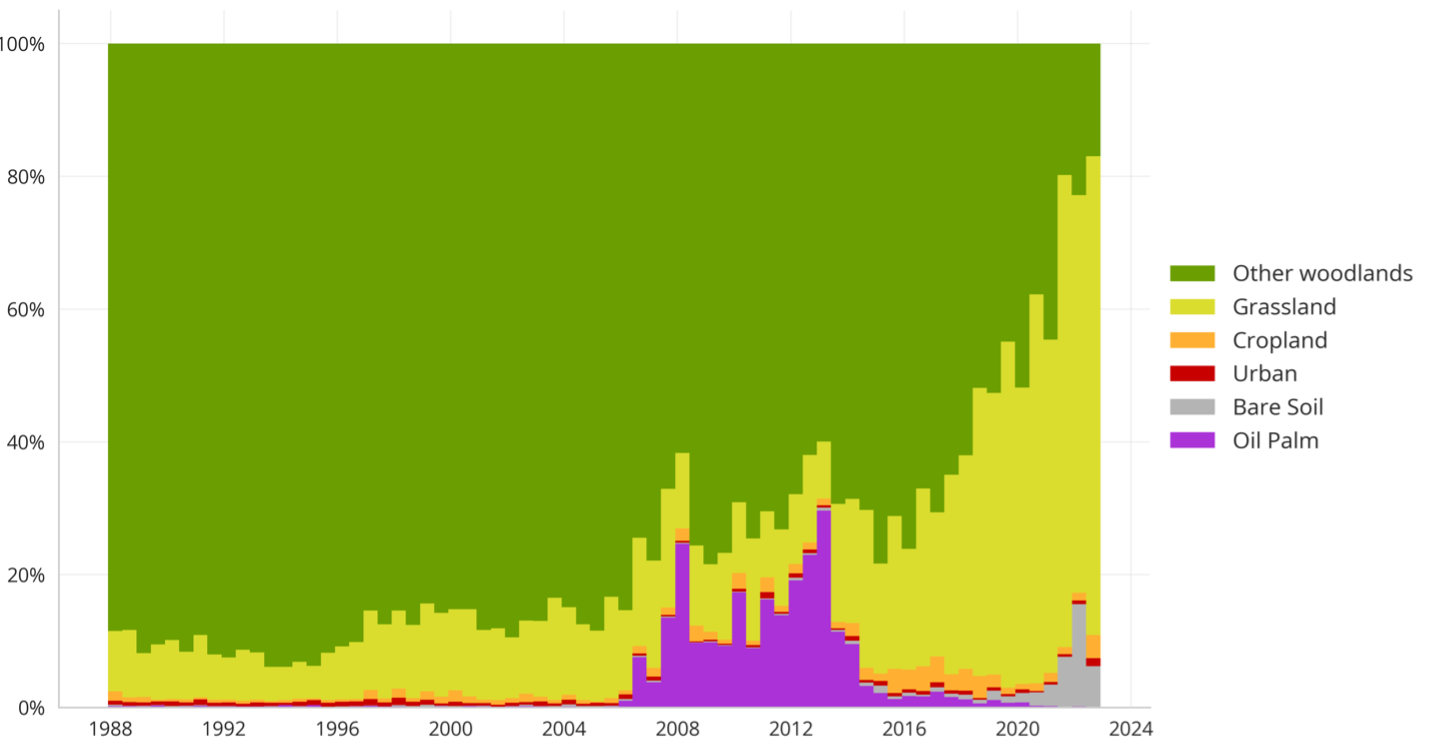
Highlighting regional efforts
On the spatial dimension, we can set targets that go below the national level: The 30-meter resolution of the new maps allow for spatially explicit performance targets, improved coordination of policy responses and a better understanding of the spatial determinants of deforestation.
In Yurimaguas, the expansion of deforestation is not only triggered by the closeness to the Yurimaguas settlement, but also to the road network. Deforestation also mostly occurs as an extension of previously deforested areas. Understanding these determinants is crucial in order to complement the design of KPIs for financial instruments with promising policy interventions.
The new data product allows us to have accurate, scalable land cover maps at the sub-annual level and with improved spatial resolution, for a longer time span than is currently available. It is now possible to have stronger KPIs with credible Monitoring, Reporting and Verification systems that can be used for better suited, more ambitious financial instruments to fight deforestation.
Read about the cloud peering technologies and the enhanced change detection algorithm underlying the new data product in the first part of this series: Peering through the clouds in the Peruvian Amazon.